-
The Spoken BNC2014 early access projects: Part 2
In January, we announced the recipients of the Spoken BNC2014 Early Access Data Grants. Over the next several months, they will use exclusive access to the first five million words of Spoken BNC2014 data to carry out a total of thirteen research projects. In this series of blogs, we are excited to share more information…
-
FireAnt has officially launched!
Laurence Anthony and Claire Hardaker first introduced FireAnt at the CL2015 conference. In their talk, Claire explained that her work with the Discourse of Online Misogyny (DOOM) project had led her to realise that when working with Twitter data, you fast encounter a large array of problems: how to easily collect data, how to arrange…
Search A Keyword
CASS Briefings
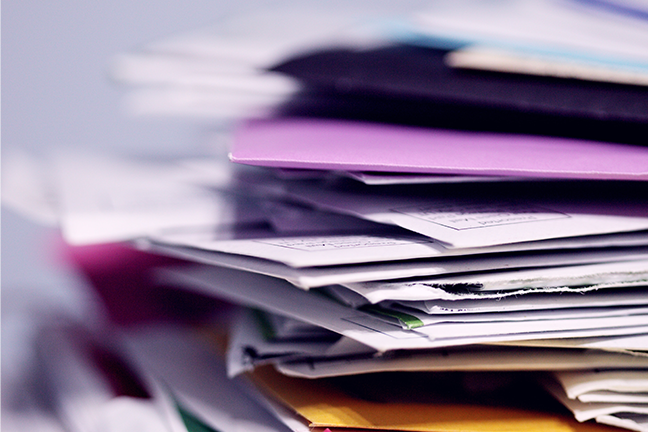
CASS: Briefings is a series of short, quick reads on the work being done at the ESRC/CASS research centre at Lancaster University, UK.
Recent Post
Tags
amanda potts brazil claire hardaker elena semino islam Islamophobia learner language metaphor metaphor in end of life care paul baker spoken BNC2014 tony mcenery Trinity Lancaster Spoken Learner Corpus trolling twitter
Categories
- Ambassadors
- Anatomy of a troll
- Applications of corpus linguistics
- Big data media analysis and the representation of urban violence in Brazil
- Blogs
- BNC2014
- Call for Papers
- CASS Affiliated Projects
- CASS Briefing
- CFIE
- Challenge Panel
- Changing Climates
- Comparable and Parallel Corpus Approaches to the Third Code
- Distressed Communities
- DOOM
- Events
- General
- Hate Speech
- Healthcare
- iCourts
- Jobs
- L2 language corpora
- Lancs Box
- learner corpora
- MA in Corpus linguistics
- Maritime Security and Piracy Discourses
- Media
- MELC
- MOOC
- News
- Newspapers
- Newspapers poverty and long-term change
- Post Event Summaries
- Research
- Spatial Humanities
- Spoken BNC2014
- Trinity
- Uncategorized
- Understanding Corporate Communications
- Urban violence